1 Global Synthetic Data Software Market Size (Value) and CAGR (2024-2033)
In 2024, the global Synthetic Data Software market was valued at USD 209.57 million, with a CAGR of 15.6% from 2024 to 2033.
Synthetic data software allows users to create artificial datasets, such as images, text, or structured data based on an initial dataset or data source. Synthetic data software lets users produce data from scratch that protects privacy-sensitive data, whilst maintaining the patterns and relationships inherent in the original dataset. Techniques for producing this synthetic data include computer-generated imagery (CGI), generative neural networks (GANs), and heuristics. Synthetic data can be a useful way for companies to build datasets in a more efficient and effective manner for the purposes of testing, machine learning model training, data validation, and more.
Figure Global Synthetic Data Software Market Size (M USD) and CAGR 2024-2033
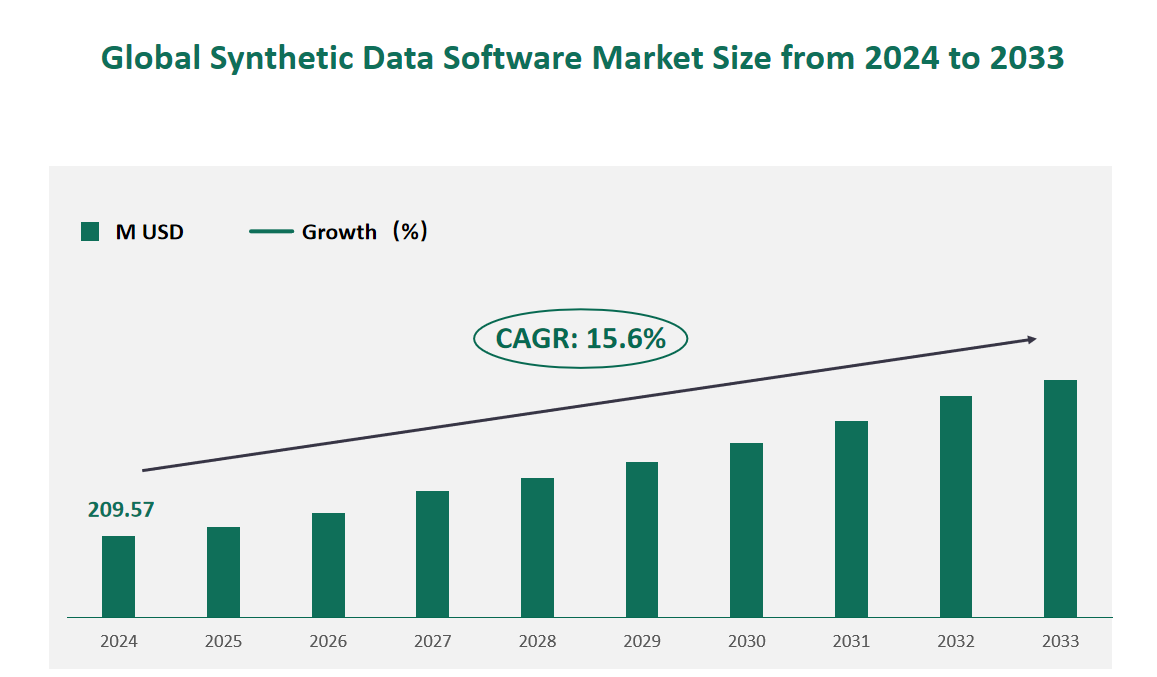
2 Synthetic Data Software Market Drivers
One of the most significant drivers of the Synthetic Data Software market is the growing emphasis on data privacy. With stringent regulations such as GDPR and CCPA, organizations are under increasing pressure to protect sensitive information. Synthetic data, which is artificially generated and not based on real individuals or events, offers a solution that allows companies to share and use data without compromising privacy. This is particularly crucial in industries like healthcare and finance, where data sensitivity is paramount. Synthetic datasets can mimic the statistical properties of real data without revealing protected information, making them an ideal substitute for real data in various applications.
Another major driver is the ability of synthetic data to enhance operational efficiency. Traditional data collection methods are often time-consuming and expensive, especially when it comes to large-scale data acquisition for machine learning and AI model training. Synthetic data, on the other hand, can be generated quickly and at a lower cost. This allows data scientists and organizations to bypass the challenges of collecting real-world data, thereby accelerating the development and deployment of AI models.
Synthetic data also offers several advantages over real data. For instance, it can be generated to include a wide range of scenarios and edge cases that are critical for training AI models but may be rare in real-world datasets. This ensures that models are better prepared to handle diverse and complex situations. Moreover, synthetic data can be automatically labeled, reducing the need for manual data labeling, which is often a labor-intensive and error-prone process. The ability to generate large volumes of high-quality, diverse data sets makes synthetic data an attractive alternative for organizations looking to enhance their data-driven capabilities.
3 Synthetic Data Software Market Challenges
One of the most significant constraints is the challenge of ensuring the quality and realism of synthetic data. While synthetic data is designed to mimic real data, there can still be differences in data distribution and nuances that may affect its applicability. Synthetic data is generated based on input data and models, meaning that any inaccuracies or biases in the input data can be perpetuated in the synthetic datasets. This can lead to suboptimal performance of machine learning models trained on synthetic data, as they may not fully capture the complexities and variability of real-world scenarios. Ensuring that synthetic data is sufficiently realistic and representative of real data is a critical challenge that requires continuous improvement in data generation techniques.
Another constraint is the technical complexity involved in generating high-quality synthetic data. The process of creating synthetic data often requires sophisticated algorithms and models, such as GANs, which can be computationally intensive and require significant expertise to implement effectively.
Evaluating the quality and effectiveness of synthetic data is another major constraint. There is currently no universal framework for assessing the quality of synthetic data, and the evaluation process often needs to be tailored to each specific dataset and use case. This can be time-consuming and resource-intensive, as it requires a thorough understanding of the intended application and the ability to compare synthetic data with real data benchmarks. The lack of standardized evaluation metrics also makes it difficult for organizations to confidently adopt synthetic data solutions, as they may struggle to determine whether the synthetic data meets their requirements.
4 Global Synthetic Data Software Market Size and Share by Type in 2024
Cloud-Based Synthetic Data Software represents a significant portion of the market, driven by its inherent flexibility and scalability. This type of software is hosted on remote servers and delivered via the internet, allowing users to access synthetic data from anywhere without the need for extensive local infrastructure. Cloud-Based solutions are particularly attractive to organizations seeking cost-effective and efficient data management.
They offer the ability to scale resources dynamically, ensuring that users can adapt to changing demands without significant upfront investment. The ease of access and the ability to integrate with existing systems further enhance the appeal of Cloud-Based Synthetic Data Software, driving its market value to 131,734 K USD in 2024.
On the other hand, On-Premises Synthetic Data Software is designed for organizations that prioritize data control and security. This type of software is installed and operated within an organization’s own data centers, providing a high level of control over data management and processing. On-Premises solutions are particularly favored by large enterprises and highly regulated industries, such as banking and healthcare, where data privacy and compliance are paramount.
These organizations often require strict governance over their data, ensuring that it remains within their internal systems and is not exposed to external environments. On-Premises solutions offer the ability to customize data management processes to meet specific organizational needs, providing a tailored approach to synthetic data generation and utilization. Despite the growing popularity of Cloud-Based solutions, On-Premises Synthetic Data Software maintains a significant market presence, with a projected value of 77,838 K USD in 2024.
Table Global Synthetic Data Software Market Size and Share by Type in 2024
Type | Market Size (K USD) 2024 | Market Share 2024 |
Cloud-Based | 131734 | 62.86% |
On-Premises | 77838 | 37.14% |
5 Global Synthetic Data Software Market Size and Share by Application in 2024
In the Retail sector, synthetic data is used to enhance customer experience, optimize supply chains, and develop personalized marketing strategies. The market value for synthetic data software in Retail is expected to reach 29,550 K USD in 2024. Synthetic data allows retailers to simulate various scenarios, predict consumer behavior, and test new products without risking sensitive customer data.
The Healthcare industry is another significant adopter of synthetic data, utilizing it for medical research, patient data privacy, and AI-driven diagnostics. The market value for Healthcare is projected to be 23,828 K USD in 2024. Synthetic data enables healthcare organizations to create realistic datasets that protect patient privacy while supporting the development of new treatments and technologies.
The IT & Telecom sector leverages synthetic data for network optimization, cybersecurity, and AI model training. With a market value of 46,483 K USD in 2024, synthetic data helps IT and telecom companies simulate complex network scenarios, test new technologies, and enhance data security without exposing real user data.
The BFSI sector, which includes banking, financial services, and insurance, is a major driver of synthetic data adoption. Synthetic data is used for fraud detection, risk assessment, and regulatory compliance. The market value for BFSI is expected to reach 65,596 K USD in 2024. This sector benefits from synthetic data’s ability to create realistic datasets that protect sensitive financial information while enabling advanced analytics and AI applications.
Table Global Synthetic Data Software Market Size and Share by Application in 2024
Application | Market Size (K USD) 2024 | Market Share 2024 |
Retail | 29550 | 14.10% |
Healthcare | 23828 | 11.37% |
IT & Telecom | 46483 | 22.18% |
BFSI | 65596 | 31.30% |
Others | 44115 | 21.05% |
6 Global Synthetic Data Software Market Size by Region in 2024
North America, led by the United States and Canada, is a dominant player in the synthetic data market. The region is projected to have a market value of 81,082 K USD in 2024. North America’s strong adoption of synthetic data is driven by advanced technological infrastructure, a high concentration of tech-savvy enterprises, and stringent data privacy regulations. The region is also at the forefront of AI and machine learning innovations, which further fuel the demand for synthetic data solutions.
Europe, with its diverse economies and robust regulatory framework, is another key market for synthetic data software. The region is expected to achieve a market value of 51,361 K USD in 2024. European countries, particularly the UK, Germany, and France, are significant adopters of synthetic data due to their focus on data privacy and compliance with regulations such as GDPR. The growth in Europe is also supported by the increasing demand for AI and machine learning applications across various industries.
The Asia-Pacific region is experiencing rapid growth in the synthetic data market, driven by the digital transformation initiatives of countries like China, Japan, and South Korea. The region is projected to have a market value of 54,846 K USD in 2024. The growth in Asia-Pacific is fueled by the increasing adoption of cloud computing, AI, and machine learning technologies. Additionally, the region’s large and growing data economy provides a fertile ground for synthetic data applications in sectors such as healthcare, finance, and IT.
Figure Global Synthetic Data Software Market Share by Region in 2024
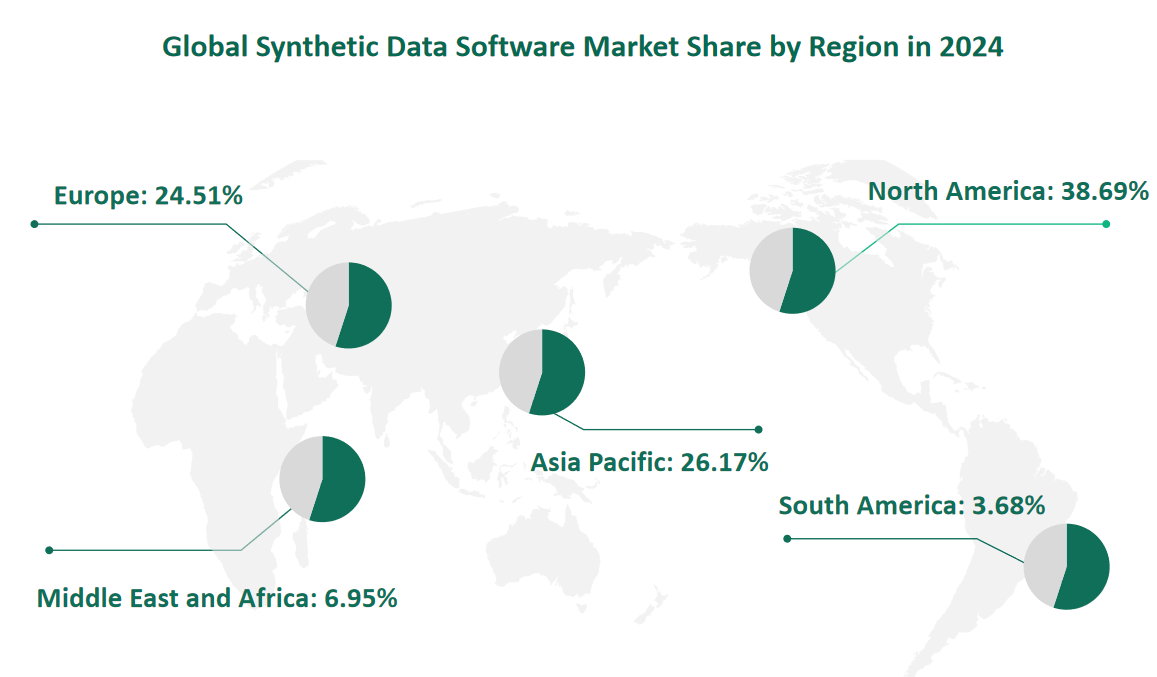
7 Major Players in Global Synthetic Data Software Market
7.1 AI.Reverie
Company Profile: AI.Reverie, established in 2017 and headquartered in the United States, is a leading provider of synthetic data solutions. The company specializes in creating realistic synthetic data for computer vision AI applications, enabling businesses across various industries to train their machine learning algorithms efficiently and accurately.
Business Overview: AI.Reverie operates as a software development company focused on generating synthetic data to support AI and machine learning initiatives. The company’s proprietary simulation platform allows users to create customized synthetic datasets that mimic real-world scenarios, ensuring high accuracy and privacy protection.
Product and Service Analysis: AI.Reverie offers a suite of simulated environments that enable users to collect datasets tailored to their deep learning models. These environments include customizable outdoor and indoor models, rural/urban environments, weather conditions, and lighting scenarios. Additionally, AI.Reverie provides dynamic objects and scenarios for training data, such as people, animals, vehicles, and inanimate objects, ensuring realistic interactions and movements. The company also offers configurable sensors for data capture, supporting various points of view and environmental conditions.
Recent Financial Performance: In the most recent year, AI.Reverie reported a market value of 4621 thousand USD and a gross margin of 50.62%.
7.2 MOSTLY AI
Company Profile: MOSTLY AI, founded in 2017 and headquartered in Austria, is a high-tech startup specializing in synthetic data generation for the financial services industry. The company’s innovative technology leverages advanced generative deep neural networks to create realistic and privacy-compliant synthetic data.
Business Overview: MOSTLY AI’s Synthetic Data Engine is designed to simulate realistic and representative synthetic data at scale. The platform automatically learns patterns, structures, and variations from existing data, ensuring that the synthetic data retains valuable information while rendering re-identification of individuals impossible. This approach enables financial institutions to use synthetic data for various internal business functions without compromising privacy.
Product and Service Analysis: MOSTLY AI’s synthetic data solutions are tailored for financial services, providing banks with flexible and privacy-compliant datasets. The company’s offerings include improving model performance by creating balanced datasets for fraud detection, speeding up proof-of-concept (PoC) projects, and providing realistic enterprise test data. MOSTLY AI’s synthetic data ensures that sensitive information remains protected while enabling efficient data sharing and analysis.
Recent Financial Performance: In the most recent year, MOSTLY AI achieved a market value of 4404 thousand USD and a gross margin of 49.48%.
7.3 CA Technologies
Company Profile: CA Technologies, established in 1976 and headquartered in the United States, is a global leader in IT management software and solutions. The company provides a wide range of products and services aimed at improving IT operations, security, and data management.
Business Overview: CA Technologies operates as an IT management software company, offering solutions in automation, cloud integration, security, service management, and test data management. The company’s Test Data Manager solution is particularly notable for its ability to create synthetic test data, ensuring efficient and secure software development processes.
Product and Service Analysis: CA Technologies’ Test Data Manager is designed to quickly locate, secure, design, create, and provision test data for efficient and cost-effective testing cycles. The solution enhances the quality of production data by filling gaps in test data coverage and supporting continuous testing requirements. Test Data Manager also ensures compliance through synthetic data creation, providing organizations with the right data at the right time to accelerate software development.
Recent Financial Performance: In the most recent year, CA Technologies reported a market value of 3904 thousand USD and a gross margin of 49.14%.